AI Transforming Modern Payment Reconciliation
AI Transforming Modern Payment Reconciliation
AI Transforming Modern Payment Reconciliation
AI Transforming Modern Payment Reconciliation
Anant Sharma
Anant Sharma
Anant Sharma
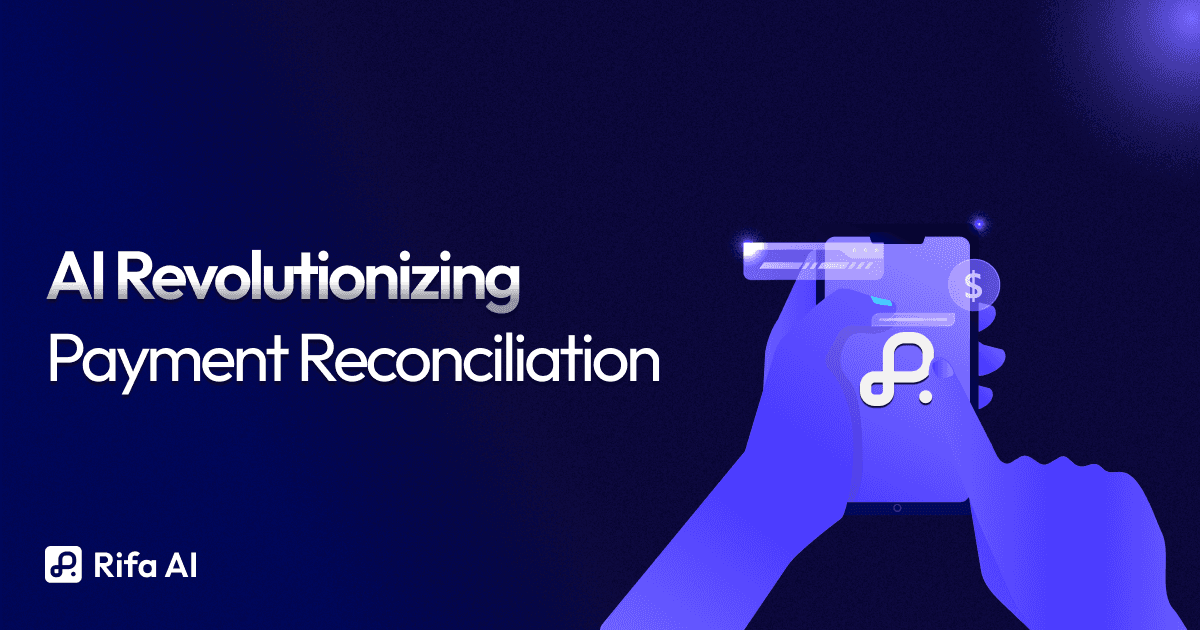
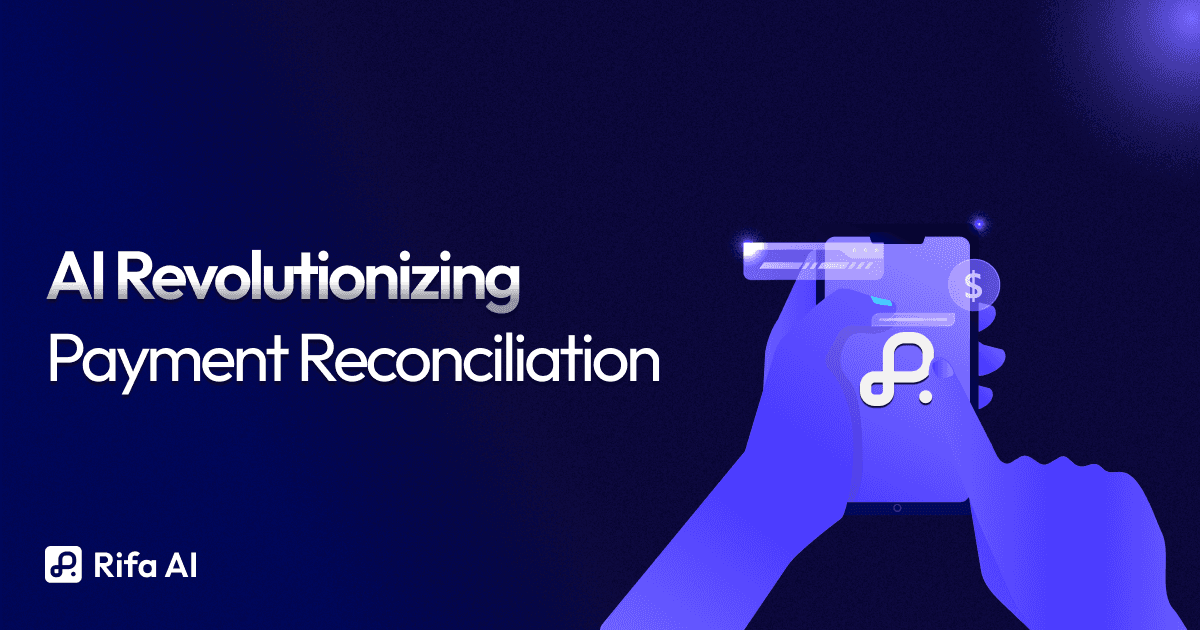
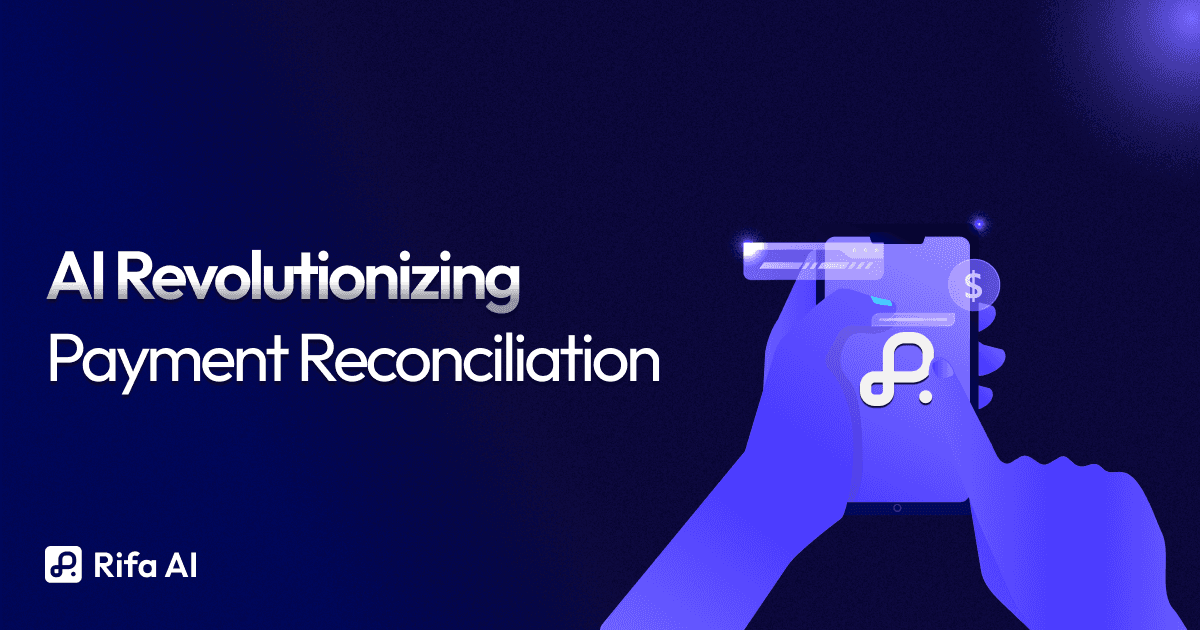
Traditionally, payment reconciliation, ensuring that payments match bank statements and invoices, has been labor-intensive and error-prone. However, AI is revolutionizing this process by streamlining operations, reducing errors, and improving efficiency.
With the growing volume of transactions, managing reconciliation has become increasingly difficult. Statista projects that the total value of digital payments worldwide will exceed $11 trillion by 2026, amplifying the need for more reliable reconciliation systems.
Manual processes can no longer keep pace with the scale and complexity of these operations. AI addresses these challenges by employing machine learning algorithms that minimize manual intervention, flag discrepancies in real time, and automate data matching.
According to a McKinsey report, businesses using AI in financial operations can save up to 33% in costs while enhancing processing speed and accuracy. AI-driven reconciliation tools not only handle discrepancies proactively but also adapt to various data formats, identify patterns, and provide predictive insights.
As financial ecosystems become increasingly interconnected, integrating AI for payment reconciliation is shifting from a competitive advantage to a necessity.
What is Payment Reconciliation?
Payment reconciliation is crucial for maintaining financial accuracy, as it ensures that your records align with bank statements and transactions across various systems. It plays an essential role in detecting discrepancies, preventing fraud, and preserving trust in financial processes.
Proper reconciliation guarantees that all financial entries—from recorded expenses to received payments—are accurate and consistent, supporting financial integrity and avoiding costly errors. For instance, it ensures that accounts payable and receivable align, facilitating accurate financial reporting and reducing compliance risks.
Problems with Conventional Reconciliation Techniques
Manual, traditional methods present significant challenges, including:
Error-Prone Processes: Manual reconciliation is time-consuming and susceptible to human error, such as incorrect data entry or timing discrepancies between statements.
Fraud Risk: The complexity and lack of real-time oversight increase the likelihood of fraudulent transactions going undetected.
Operational Bottlenecks: High data volumes and a lack of system integration slow down processes and reduce efficiency.
Ineffective Decision-Making: Conventional systems often fail to relate anomalies to broader financial patterns, limiting the effectiveness of decision-making.
Adopting AI for payment reconciliation becomes more efficient, accurate, and secure, freeing up resources for strategic initiatives.
Achieve unmatched accuracy, identify disparities instantly, and streamline processes for faster, more reliable financial management with Rifa AI.
Agent Time Savings: Automate repetitive tasks, allowing your team to focus on more strategic projects.
Quick Deployment: Implement and go live in days without the need for developer or API support.
2x Improved Payment Conversions: Boost customer engagement and improve payment success rates.
40% Faster Debt Recovery: Accelerate collection cycles and increase cash flow with AI-driven negotiation tools.
99% Accuracy: AI systems ensure precision in every interaction, minimizing errors.
Reduce Operational Costs by Up to 70%: Streamline processes to improve efficiency.
We have the solutions you need to improve data integrity in reconciliation to achieve faster, more accurate results. Improve your reconciliation accuracy with Rifa AI—contact us today!
To appreciate the impact of AI, we must first examine how traditional reconciliation processes operate. By learning the steps involved and identifying inherent inefficiencies, we can better grasp the necessity for innovation in this space.
Traditional Payment Reconciliation Processes
Traditional methods, relying heavily on manual intervention, are resource-intensive, slow, and prone to errors. Here's a look at how AI is transforming these outdated practices:
1. Manual Data Entry
Traditional payment reconciliation requires extensive manual data entry—updating records, entering transaction details, and cross-checking statements, which is both time-consuming and error-prone. AI helps by automating data collection through OCR and NLP.
For example, AI can instantly update financial records by scanning bank statements and invoices, extracting relevant information, and populating it into the system.
2. Transaction Matching
Matching transactions to accounts or invoices is another time-consuming task. Manually comparing bank statements with internal records is common in traditional methods, and handling multiple currencies or payment gateways makes it even more challenging.
AI reduces mismatches by using machine learning to match transactions based on patterns.
Using ML, Rifa AI matches transactions to accounts or invoices with precision, reducing mismatches and improving reconciliation across multiple currencies or payment gateways.
3. Identifying Discrepancies
Traditional methods require going through each record line by line to detect discrepancies—a labor-intensive process prone to error. AI excels in real-time discrepancy detection by quickly and accurately flagging issues such as duplicate payments, missing entries, or mismatched amounts.
For example, AI-powered systems like BlackLine detect and resolve deviations promptly, ensuring accurate financial reporting.
4. Drawbacks of Conventional Approaches
Conventional methods have several notable disadvantages, including human errors—simple numeric entry mistakes or omissions that can cause reconciliation issues.
Manually reconciling large transaction volumes can lead to delays in reporting. AI minimizes these drawbacks by learning from previous reconciliations and continuously improving accuracy.
For example, Xero's AI-powered reconciliation software speeds up the process and reduces human intervention, allowing more time for strategic financial planning.
Switching to AI-powered reconciliation tools can significantly reduce errors and inefficiencies, leading to a more effective and streamlined payment management system.
With Rifa AI, you can maximize your collections process's potential by doubling payment conversions and reducing collection cycles by up to 40%. Our AI-powered solutions optimize processes, cut agent time by 50%, and ensure full compliance with industry standards. Start with Rifa AI today to upgrade your payment reconciliation and debt recovery processes.
As businesses seek faster and more reliable solutions, AI emerges as a transformative force.
The following section highlights how AI augments reconciliation through task automation, heightened accuracy, and real-time transaction processing, fundamentally changing the way businesses manage their finances.
Role of AI for Payment Reconciliation
Artificial intelligence is redefining contemporary payment reconciliation by changing the way we handle financial operations. Here are three important areas where AI's capabilities are valuable:
1. Automating Tedious Tasks
AI automates labor-intensive tasks like variance reconciliation, error detection, and data matching. Traditional procedures often required manual input, which was slow and prone to errors. AI-driven platforms, like those integrating RPA and ML, efficiently handle tasks such as transaction matching and report generation.
For example, reconciliation systems from Akira AI save time and money while ensuring accuracy by automating data collection from various sources and managing discrepancies with minimal human involvement.
2. Transaction Processing in Real-Time
As transactions occur, real-time systems quickly compare and analyze them, identifying discrepancies and enabling prompt corrective actions. This is crucial in sectors like banking and e-commerce, which handle high volumes of transactions.
For example, Rifa AI empowers businesses with real-time reconciliation capabilities, which is crucial for sectors like banking and e-commerce. By integrating ML and generative AI models, Rifa AI enables proactive fraud detection, monitors transaction patterns continuously, and allows instant corrective actions.
3. Machine Learning for Improved Accuracy
ML algorithms improve reconciliation accuracy by identifying patterns and anomalies in large datasets. These systems continuously improve by learning from past reconciliations, resulting in fewer inconsistencies over time.
For example, Modern Treasury uses AI to suggest customized reconciliation rules that adapt to specific financial data, achieving near-perfect item-level accuracy. This reduces human error and ensures compliance with financial regulations.
These advancements show how AI is essential for modern payment reconciliation efforts. It not only improves operational efficiency but also strengthens the accuracy and reliability of financial systems.
The real power of AI lies in its innovative approaches. From OCR for extracting critical details to NLP for advanced data matching, the following section outlines the key methods AI uses to optimize reconciliation procedures.
AI Techniques Improving Reconciliation
Payment reconciliation procedures are changing due to AI, making them faster, more accurate, and requiring fewer resources. AI-driven techniques such as NLP, OCR, and Pattern Recognition with Anomaly Detection ensure effective transaction matching and error detection, reducing financial discrepancies and saving time.
1. Pattern Recognition and Anomaly Detection:
ML models trained on transaction data enable real-time pattern recognition and anomaly detection. These tools flag outliers that might indicate errors or fraud, such as unusual transaction amounts or payment patterns.
Using advanced ML models, Rifa AI identifies transaction patterns and detects anomalies in real time. Techniques similar to isolation forests or Z-scores are employed to flag outliers—such as irregular payment amounts—enabling proactive fraud detection and resolution.
2. Using NLP for Advanced Data Matching
AI-powered NLP can match complex financial data from diverse sources, interpreting unstructured data like invoices, emails, and payment descriptions.
For instance, Named Entity Recognition (NER)-trained machine learning models can identify key components such as vendor names, invoice numbers, and payment amounts.
This technology improves reconciliation accuracy, especially when dealing with non-standard data formats like handwritten notes or documents in multiple languages, reducing the need for manual intervention.
3. OCR for Data Extraction from Documents:
OCR is crucial for extracting data from digital or physical documents like invoices and receipts. Once extracted, the text is integrated with other AI systems for validation and classification.
This is particularly beneficial for large organizations handling invoices from multiple sources, as OCR converts invoice images into machine-readable formats for easier examination of inconsistencies.
This automation reduces human error and ensures high data integrity during reconciliation.
By ensuring accuracy, transparency, and regulatory compliance, these AI-driven innovations improve data security and streamline reconciliation processes. Implementing these technologies reduces the risks associated with manual processing and accelerates reconciliation cycles.
Rifa AI guarantees precision, effectiveness, and error-free financial transactions through AI-driven methods such as NLP, OCR, and Pattern Recognition with Anomaly Detection. OCR enables smooth extraction and validation of data from documents, minimizing human error, while Rifa AI improves data matching by using NLP to handle unstructured data like invoices, emails, and payment descriptions.
Get Rifa AI today to learn how our smart solutions can improve your performance and optimize your workflow.
Incorporating AI does not merely modernize reconciliation; it redefines it. Here, we will explore the primary benefits, including increased speed, boosted accuracy, and advanced fraud detection capabilities, making a compelling case for adoption.
Benefits of AI for Payment Reconciliation
AI for payment reconciliation significantly increases accuracy, efficiency, and security. Here’s how it benefits:
1. Improved Discrepancy Resolution Accuracy
Reconciliation errors, such as discrepancies between invoices and payments, can result in significant financial losses and are time-consuming to resolve. AI systems accurately cross-reference data to minimize these errors.
Using ML algorithms, they flag inconsistencies, identify patterns, and suggest corrective actions. AI can detect anomalies, such as payments with incorrect reference numbers, and alert you to make necessary adjustments, ensuring precise financial reporting.
2. Increased Performance and Speed
AI-powered systems can handle large volumes of data at speeds unmatched by human labor. By automating repetitive tasks such as matching receipts, payments, and invoices, AI frees up time for more strategic decision-making.
For example, an AI system can match hundreds of transactions across multiple accounts in seconds, reducing the time required for end-of-month reconciliations from days to just hours.
Rifa AI automates the reconciliation of large data volumes, reducing processing time. Its advanced ML algorithms can handle tasks like matching receipts, payments, and invoices across multiple accounts within seconds.
3. Better Fraud Detection
AI excels at identifying fraudulent activity by analyzing transaction patterns and spotting irregularities in real-time. Unlike manual systems, which might miss small anomalies, AI is highly effective in detecting suspicious transactions.
For example, AI can trigger alerts if an account exhibits unusual payment activity, such as frequent chargebacks or excessive refunds, prompting immediate action to prevent fraud. Payment networks like Mastercard leverage AI to improve security, giving both consumers and businesses peace of mind.
AI for payment reconciliation boosts speed, accuracy, and security. With AI, reconciliation processes become smoother and more reliable, transforming how businesses manage daily transactions and handle complex financial data.
Transitioning to AI-powered reconciliation requires thoughtful planning and implementation.
The next section guides on selecting the right tools, preparing data effectively, and customizing AI solutions to meet specific business needs.
Implementing AI Solutions in Payment Reconciliation
AI for payment reconciliation improves financial transaction management, scalability, accuracy, and efficiency. Implementing AI requires a structured approach that involves selecting the right tools, preparing and analyzing data, and customizing the solution to meet specific business needs.
1. Selecting the Correct AI Tools
The first step is evaluating AI tools based on how well they integrate with your existing systems and reconciliation processes. Look for platforms that offer features like anomaly detection, automated matching, and integration with ERP systems.
These systems support a variety of data sources, including emails, invoices, and remittance advice, and ensure compatibility with multiple formats without requiring major IT changes.
2. Preparing and Analyzing Data
AI systems require clean, consistent, and organized data to function effectively. For instance, generative AI excels at identifying inconsistencies, improving data quality, and converting unstructured payment data into standardized formats.
Data normalization and cleansing can speed up the reconciliation process and reduce error rates. AI-powered tools with ingestion capabilities can automate this step, saving time and improving overall data accuracy.
For example, Rifa AI automates data ingestion, normalization, and cleansing processes, ensuring data consistency and accuracy. Its NLP and ML capabilities standardize unstructured payment data, significantly speeding up reconciliation while reducing error rates.
3. Customization and Tailoring to Specific Requirements
AI solutions need to be tailored to fit your company’s specific processes. Customization involves training the AI system using historical data to recognize trends, regulations, and anomalies unique to your transactions.
For example, platforms like Citi Smart Match become more accurate over time in resolving mismatched items by learning from manual interventions. With proper training, these systems can predict reconciliation problems, detect fraudulent activities, and adapt to new payment scenarios.
AI for payment reconciliation not only boosts productivity but also provides valuable insights that help businesses make better financial decisions and position themselves for future growth.
Look no further than Rifa AI to unlock the full potential of AI-driven payment reconciliation. We eliminate the need for complicated API integrations by integrating with your current systems, ensuring smooth, round-the-clock operations.
Rifa AI lowers operating costs by up to 70%. Get Rifa AI today, and ensure compliance and minimize the risk of inconsistencies while automating repetitive tasks, freeing up your team’s time to focus on strategic decision-making.
Emerging trends like predictive analytics and blockchain integration are shaping the future of financial management. The next section examines the evolving landscape and how businesses can prepare for upcoming advancements.
Future Trends in AI for Payment Reconciliation
AI for payment reconciliation betters financial processes, security, accuracy, and efficiency. New trends such as predictive analytics, blockchain integration, and customized solutions are shaping the future of reconciliation.
1. Integration of Blockchain and AI
The integration of blockchain and AI brings greater accuracy and transparency to reconciliation. Blockchain, as a secure and immutable ledger, ensures the authenticity of transaction records.
AI improves this by analyzing real-time data from blockchain networks to detect inconsistencies, irregularities, or potential fraud.
For instance, companies can use blockchain to create shared financial ledgers accessible to all parties, while AI quickly identifies anomalies.
2. Planning with Predictive Analytics
By analyzing historical data and identifying trends, these tools predict potential inconsistencies or mismatches.
Rifa AI’s predictive analytics capabilities empower businesses to forecast potential reconciliation challenges based on historical data.
Identifying transaction trends and cash flow patterns helps businesses proactively address issues, ensuring smoother operations. Rifa AI can optimize payment schedules during peak seasons, reducing errors and delays in transaction processing.
3. Greater Emphasis on Personalized Solutions
Focus enables customized reconciliation solutions tailored to a company’s specific needs. Advanced ML algorithms adjust to distinct transaction patterns and recommend strategies for improvement. This is especially beneficial for small and medium-sized businesses dealing with various payment systems.
AI-powered personalized dashboards highlight key reconciliation metrics, helping businesses focus on areas requiring attention. Moreover, AI solutions easily integrate with existing accounting software, providing a user-friendly, customized experience.
By adopting these trends, businesses can process and position themselves for sustained growth in a competitive market.
Conclusion
By automating manual processes, AI reduces the likelihood of human error and speeds up the identification of inconsistencies. AI systems leveraging machine learning can adapt to changing patterns over time, improving their accuracy incrementally.
The AI-driven fraud detection technology demonstrates how AI can streamline operations while mitigating risks. This system monitors transactions in real-time, identifying potential fraudulent activities with high precision, thereby protecting both businesses and customers. Implementing AI for payment reconciliation ensures compliance, manages increasing volumes efficiently, and maintains high levels of customer satisfaction.
To future-proof financial operations and achieve unprecedented efficiency, businesses should explore and adopt AI-driven solutions for payment reconciliation.
See how Rifa AI can transform your payment reconciliation efforts, collections process, and reduce expenses. Rifa AI boosts accuracy by up to 99% while minimizing human error, accelerating fraud detection, and ensuring compliance by automating manual processes.
Due to its integration into existing systems, Rifa AI can be deployed in just a matter of days and can reduce operating costs by up to 70% without the need for an API. Schedule a demo with Rifa AI and discover how we can boost your company’s efficiency and security by streamlining payment and collection procedures.
Traditionally, payment reconciliation, ensuring that payments match bank statements and invoices, has been labor-intensive and error-prone. However, AI is revolutionizing this process by streamlining operations, reducing errors, and improving efficiency.
With the growing volume of transactions, managing reconciliation has become increasingly difficult. Statista projects that the total value of digital payments worldwide will exceed $11 trillion by 2026, amplifying the need for more reliable reconciliation systems.
Manual processes can no longer keep pace with the scale and complexity of these operations. AI addresses these challenges by employing machine learning algorithms that minimize manual intervention, flag discrepancies in real time, and automate data matching.
According to a McKinsey report, businesses using AI in financial operations can save up to 33% in costs while enhancing processing speed and accuracy. AI-driven reconciliation tools not only handle discrepancies proactively but also adapt to various data formats, identify patterns, and provide predictive insights.
As financial ecosystems become increasingly interconnected, integrating AI for payment reconciliation is shifting from a competitive advantage to a necessity.
What is Payment Reconciliation?
Payment reconciliation is crucial for maintaining financial accuracy, as it ensures that your records align with bank statements and transactions across various systems. It plays an essential role in detecting discrepancies, preventing fraud, and preserving trust in financial processes.
Proper reconciliation guarantees that all financial entries—from recorded expenses to received payments—are accurate and consistent, supporting financial integrity and avoiding costly errors. For instance, it ensures that accounts payable and receivable align, facilitating accurate financial reporting and reducing compliance risks.
Problems with Conventional Reconciliation Techniques
Manual, traditional methods present significant challenges, including:
Error-Prone Processes: Manual reconciliation is time-consuming and susceptible to human error, such as incorrect data entry or timing discrepancies between statements.
Fraud Risk: The complexity and lack of real-time oversight increase the likelihood of fraudulent transactions going undetected.
Operational Bottlenecks: High data volumes and a lack of system integration slow down processes and reduce efficiency.
Ineffective Decision-Making: Conventional systems often fail to relate anomalies to broader financial patterns, limiting the effectiveness of decision-making.
Adopting AI for payment reconciliation becomes more efficient, accurate, and secure, freeing up resources for strategic initiatives.
Achieve unmatched accuracy, identify disparities instantly, and streamline processes for faster, more reliable financial management with Rifa AI.
Agent Time Savings: Automate repetitive tasks, allowing your team to focus on more strategic projects.
Quick Deployment: Implement and go live in days without the need for developer or API support.
2x Improved Payment Conversions: Boost customer engagement and improve payment success rates.
40% Faster Debt Recovery: Accelerate collection cycles and increase cash flow with AI-driven negotiation tools.
99% Accuracy: AI systems ensure precision in every interaction, minimizing errors.
Reduce Operational Costs by Up to 70%: Streamline processes to improve efficiency.
We have the solutions you need to improve data integrity in reconciliation to achieve faster, more accurate results. Improve your reconciliation accuracy with Rifa AI—contact us today!
To appreciate the impact of AI, we must first examine how traditional reconciliation processes operate. By learning the steps involved and identifying inherent inefficiencies, we can better grasp the necessity for innovation in this space.
Traditional Payment Reconciliation Processes
Traditional methods, relying heavily on manual intervention, are resource-intensive, slow, and prone to errors. Here's a look at how AI is transforming these outdated practices:
1. Manual Data Entry
Traditional payment reconciliation requires extensive manual data entry—updating records, entering transaction details, and cross-checking statements, which is both time-consuming and error-prone. AI helps by automating data collection through OCR and NLP.
For example, AI can instantly update financial records by scanning bank statements and invoices, extracting relevant information, and populating it into the system.
2. Transaction Matching
Matching transactions to accounts or invoices is another time-consuming task. Manually comparing bank statements with internal records is common in traditional methods, and handling multiple currencies or payment gateways makes it even more challenging.
AI reduces mismatches by using machine learning to match transactions based on patterns.
Using ML, Rifa AI matches transactions to accounts or invoices with precision, reducing mismatches and improving reconciliation across multiple currencies or payment gateways.
3. Identifying Discrepancies
Traditional methods require going through each record line by line to detect discrepancies—a labor-intensive process prone to error. AI excels in real-time discrepancy detection by quickly and accurately flagging issues such as duplicate payments, missing entries, or mismatched amounts.
For example, AI-powered systems like BlackLine detect and resolve deviations promptly, ensuring accurate financial reporting.
4. Drawbacks of Conventional Approaches
Conventional methods have several notable disadvantages, including human errors—simple numeric entry mistakes or omissions that can cause reconciliation issues.
Manually reconciling large transaction volumes can lead to delays in reporting. AI minimizes these drawbacks by learning from previous reconciliations and continuously improving accuracy.
For example, Xero's AI-powered reconciliation software speeds up the process and reduces human intervention, allowing more time for strategic financial planning.
Switching to AI-powered reconciliation tools can significantly reduce errors and inefficiencies, leading to a more effective and streamlined payment management system.
With Rifa AI, you can maximize your collections process's potential by doubling payment conversions and reducing collection cycles by up to 40%. Our AI-powered solutions optimize processes, cut agent time by 50%, and ensure full compliance with industry standards. Start with Rifa AI today to upgrade your payment reconciliation and debt recovery processes.
As businesses seek faster and more reliable solutions, AI emerges as a transformative force.
The following section highlights how AI augments reconciliation through task automation, heightened accuracy, and real-time transaction processing, fundamentally changing the way businesses manage their finances.
Role of AI for Payment Reconciliation
Artificial intelligence is redefining contemporary payment reconciliation by changing the way we handle financial operations. Here are three important areas where AI's capabilities are valuable:
1. Automating Tedious Tasks
AI automates labor-intensive tasks like variance reconciliation, error detection, and data matching. Traditional procedures often required manual input, which was slow and prone to errors. AI-driven platforms, like those integrating RPA and ML, efficiently handle tasks such as transaction matching and report generation.
For example, reconciliation systems from Akira AI save time and money while ensuring accuracy by automating data collection from various sources and managing discrepancies with minimal human involvement.
2. Transaction Processing in Real-Time
As transactions occur, real-time systems quickly compare and analyze them, identifying discrepancies and enabling prompt corrective actions. This is crucial in sectors like banking and e-commerce, which handle high volumes of transactions.
For example, Rifa AI empowers businesses with real-time reconciliation capabilities, which is crucial for sectors like banking and e-commerce. By integrating ML and generative AI models, Rifa AI enables proactive fraud detection, monitors transaction patterns continuously, and allows instant corrective actions.
3. Machine Learning for Improved Accuracy
ML algorithms improve reconciliation accuracy by identifying patterns and anomalies in large datasets. These systems continuously improve by learning from past reconciliations, resulting in fewer inconsistencies over time.
For example, Modern Treasury uses AI to suggest customized reconciliation rules that adapt to specific financial data, achieving near-perfect item-level accuracy. This reduces human error and ensures compliance with financial regulations.
These advancements show how AI is essential for modern payment reconciliation efforts. It not only improves operational efficiency but also strengthens the accuracy and reliability of financial systems.
The real power of AI lies in its innovative approaches. From OCR for extracting critical details to NLP for advanced data matching, the following section outlines the key methods AI uses to optimize reconciliation procedures.
AI Techniques Improving Reconciliation
Payment reconciliation procedures are changing due to AI, making them faster, more accurate, and requiring fewer resources. AI-driven techniques such as NLP, OCR, and Pattern Recognition with Anomaly Detection ensure effective transaction matching and error detection, reducing financial discrepancies and saving time.
1. Pattern Recognition and Anomaly Detection:
ML models trained on transaction data enable real-time pattern recognition and anomaly detection. These tools flag outliers that might indicate errors or fraud, such as unusual transaction amounts or payment patterns.
Using advanced ML models, Rifa AI identifies transaction patterns and detects anomalies in real time. Techniques similar to isolation forests or Z-scores are employed to flag outliers—such as irregular payment amounts—enabling proactive fraud detection and resolution.
2. Using NLP for Advanced Data Matching
AI-powered NLP can match complex financial data from diverse sources, interpreting unstructured data like invoices, emails, and payment descriptions.
For instance, Named Entity Recognition (NER)-trained machine learning models can identify key components such as vendor names, invoice numbers, and payment amounts.
This technology improves reconciliation accuracy, especially when dealing with non-standard data formats like handwritten notes or documents in multiple languages, reducing the need for manual intervention.
3. OCR for Data Extraction from Documents:
OCR is crucial for extracting data from digital or physical documents like invoices and receipts. Once extracted, the text is integrated with other AI systems for validation and classification.
This is particularly beneficial for large organizations handling invoices from multiple sources, as OCR converts invoice images into machine-readable formats for easier examination of inconsistencies.
This automation reduces human error and ensures high data integrity during reconciliation.
By ensuring accuracy, transparency, and regulatory compliance, these AI-driven innovations improve data security and streamline reconciliation processes. Implementing these technologies reduces the risks associated with manual processing and accelerates reconciliation cycles.
Rifa AI guarantees precision, effectiveness, and error-free financial transactions through AI-driven methods such as NLP, OCR, and Pattern Recognition with Anomaly Detection. OCR enables smooth extraction and validation of data from documents, minimizing human error, while Rifa AI improves data matching by using NLP to handle unstructured data like invoices, emails, and payment descriptions.
Get Rifa AI today to learn how our smart solutions can improve your performance and optimize your workflow.
Incorporating AI does not merely modernize reconciliation; it redefines it. Here, we will explore the primary benefits, including increased speed, boosted accuracy, and advanced fraud detection capabilities, making a compelling case for adoption.
Benefits of AI for Payment Reconciliation
AI for payment reconciliation significantly increases accuracy, efficiency, and security. Here’s how it benefits:
1. Improved Discrepancy Resolution Accuracy
Reconciliation errors, such as discrepancies between invoices and payments, can result in significant financial losses and are time-consuming to resolve. AI systems accurately cross-reference data to minimize these errors.
Using ML algorithms, they flag inconsistencies, identify patterns, and suggest corrective actions. AI can detect anomalies, such as payments with incorrect reference numbers, and alert you to make necessary adjustments, ensuring precise financial reporting.
2. Increased Performance and Speed
AI-powered systems can handle large volumes of data at speeds unmatched by human labor. By automating repetitive tasks such as matching receipts, payments, and invoices, AI frees up time for more strategic decision-making.
For example, an AI system can match hundreds of transactions across multiple accounts in seconds, reducing the time required for end-of-month reconciliations from days to just hours.
Rifa AI automates the reconciliation of large data volumes, reducing processing time. Its advanced ML algorithms can handle tasks like matching receipts, payments, and invoices across multiple accounts within seconds.
3. Better Fraud Detection
AI excels at identifying fraudulent activity by analyzing transaction patterns and spotting irregularities in real-time. Unlike manual systems, which might miss small anomalies, AI is highly effective in detecting suspicious transactions.
For example, AI can trigger alerts if an account exhibits unusual payment activity, such as frequent chargebacks or excessive refunds, prompting immediate action to prevent fraud. Payment networks like Mastercard leverage AI to improve security, giving both consumers and businesses peace of mind.
AI for payment reconciliation boosts speed, accuracy, and security. With AI, reconciliation processes become smoother and more reliable, transforming how businesses manage daily transactions and handle complex financial data.
Transitioning to AI-powered reconciliation requires thoughtful planning and implementation.
The next section guides on selecting the right tools, preparing data effectively, and customizing AI solutions to meet specific business needs.
Implementing AI Solutions in Payment Reconciliation
AI for payment reconciliation improves financial transaction management, scalability, accuracy, and efficiency. Implementing AI requires a structured approach that involves selecting the right tools, preparing and analyzing data, and customizing the solution to meet specific business needs.
1. Selecting the Correct AI Tools
The first step is evaluating AI tools based on how well they integrate with your existing systems and reconciliation processes. Look for platforms that offer features like anomaly detection, automated matching, and integration with ERP systems.
These systems support a variety of data sources, including emails, invoices, and remittance advice, and ensure compatibility with multiple formats without requiring major IT changes.
2. Preparing and Analyzing Data
AI systems require clean, consistent, and organized data to function effectively. For instance, generative AI excels at identifying inconsistencies, improving data quality, and converting unstructured payment data into standardized formats.
Data normalization and cleansing can speed up the reconciliation process and reduce error rates. AI-powered tools with ingestion capabilities can automate this step, saving time and improving overall data accuracy.
For example, Rifa AI automates data ingestion, normalization, and cleansing processes, ensuring data consistency and accuracy. Its NLP and ML capabilities standardize unstructured payment data, significantly speeding up reconciliation while reducing error rates.
3. Customization and Tailoring to Specific Requirements
AI solutions need to be tailored to fit your company’s specific processes. Customization involves training the AI system using historical data to recognize trends, regulations, and anomalies unique to your transactions.
For example, platforms like Citi Smart Match become more accurate over time in resolving mismatched items by learning from manual interventions. With proper training, these systems can predict reconciliation problems, detect fraudulent activities, and adapt to new payment scenarios.
AI for payment reconciliation not only boosts productivity but also provides valuable insights that help businesses make better financial decisions and position themselves for future growth.
Look no further than Rifa AI to unlock the full potential of AI-driven payment reconciliation. We eliminate the need for complicated API integrations by integrating with your current systems, ensuring smooth, round-the-clock operations.
Rifa AI lowers operating costs by up to 70%. Get Rifa AI today, and ensure compliance and minimize the risk of inconsistencies while automating repetitive tasks, freeing up your team’s time to focus on strategic decision-making.
Emerging trends like predictive analytics and blockchain integration are shaping the future of financial management. The next section examines the evolving landscape and how businesses can prepare for upcoming advancements.
Future Trends in AI for Payment Reconciliation
AI for payment reconciliation betters financial processes, security, accuracy, and efficiency. New trends such as predictive analytics, blockchain integration, and customized solutions are shaping the future of reconciliation.
1. Integration of Blockchain and AI
The integration of blockchain and AI brings greater accuracy and transparency to reconciliation. Blockchain, as a secure and immutable ledger, ensures the authenticity of transaction records.
AI improves this by analyzing real-time data from blockchain networks to detect inconsistencies, irregularities, or potential fraud.
For instance, companies can use blockchain to create shared financial ledgers accessible to all parties, while AI quickly identifies anomalies.
2. Planning with Predictive Analytics
By analyzing historical data and identifying trends, these tools predict potential inconsistencies or mismatches.
Rifa AI’s predictive analytics capabilities empower businesses to forecast potential reconciliation challenges based on historical data.
Identifying transaction trends and cash flow patterns helps businesses proactively address issues, ensuring smoother operations. Rifa AI can optimize payment schedules during peak seasons, reducing errors and delays in transaction processing.
3. Greater Emphasis on Personalized Solutions
Focus enables customized reconciliation solutions tailored to a company’s specific needs. Advanced ML algorithms adjust to distinct transaction patterns and recommend strategies for improvement. This is especially beneficial for small and medium-sized businesses dealing with various payment systems.
AI-powered personalized dashboards highlight key reconciliation metrics, helping businesses focus on areas requiring attention. Moreover, AI solutions easily integrate with existing accounting software, providing a user-friendly, customized experience.
By adopting these trends, businesses can process and position themselves for sustained growth in a competitive market.
Conclusion
By automating manual processes, AI reduces the likelihood of human error and speeds up the identification of inconsistencies. AI systems leveraging machine learning can adapt to changing patterns over time, improving their accuracy incrementally.
The AI-driven fraud detection technology demonstrates how AI can streamline operations while mitigating risks. This system monitors transactions in real-time, identifying potential fraudulent activities with high precision, thereby protecting both businesses and customers. Implementing AI for payment reconciliation ensures compliance, manages increasing volumes efficiently, and maintains high levels of customer satisfaction.
To future-proof financial operations and achieve unprecedented efficiency, businesses should explore and adopt AI-driven solutions for payment reconciliation.
See how Rifa AI can transform your payment reconciliation efforts, collections process, and reduce expenses. Rifa AI boosts accuracy by up to 99% while minimizing human error, accelerating fraud detection, and ensuring compliance by automating manual processes.
Due to its integration into existing systems, Rifa AI can be deployed in just a matter of days and can reduce operating costs by up to 70% without the need for an API. Schedule a demo with Rifa AI and discover how we can boost your company’s efficiency and security by streamlining payment and collection procedures.
Dec 17, 2024
Dec 17, 2024
Dec 17, 2024